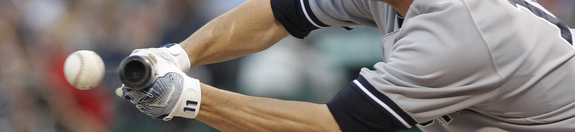
Baseball is a game without an official clock. In its stead, the 27 outs each team receives serve as the timekeeper, pushing each game to an inevitable conclusion. Avoiding those outs has become the name of the game over the last ten years, and one of the strategic moves that has come under fire due to this philosophy is the bunt. The sacrifice bunt draws a team one out closer to the end of the game without greatly increasing the chances of a run scoring. A look at run expectancy tables, which tell us how many runs are expected given a particular situation, confirms that bunting usually decreases the number of runs expected to score. While there are a few situations where a bunt is actually the statistically prudent move, on balance it is seen as the misused weapon of weaker, backwards-thinking managers, and is the hobgoblin of sabermetricians everywhere.
All that said, there is at least one study that suggests that managers tend to outperform run expectancy tables when it comes to bunting. This means that on average, managers have a reasonably good sense of the moment and of context, and they bunt in situations where it will produce more runs than one might expect given the post-bunt base/out status. While the numbers still suggest that these bunts decrease run expectancy, it is illuminating and encouraging to see that managers are utilizing the bunt reasonably efficiently.
All of this brings us to the manager of the local nine. One of the most common complaints about Joe Girardi’s managing is that he bunts too frequently, playing for one run with an offense that can put up a crooked number in a hurry. I thought it would be instructive to look at every Yankee sacrifice bunt in 2011 to see how many runs Girardi actually cost his club with his small ball sensibilities. I broke the bunts down by player and then calculated three numbers:
- Expected runs before the bunt. This number tells us how many runs were expected to score given the base/out situation prior to Girardi working his managerial magic.
- Expected runs after the bunt. This tells us how many theoretical runs the bunt “cost” the club.
- Actual runs. This should tell us how Girardi’s move actually worked out.
Now, a few caveats.
- Run expectancy is not perfect. It does not account for the score or the quality of offense or opponent, nor does it account for the skills of the hitter at the plate. However, it is a reasonable estimate of how the game has been impacted by a move, and I’ve broken things down by hitter so you can mentally adjust your evaluation based on the quality of the batter.
- This study does not include the attempted bunts that failed and caused batters to fall behind in the count. However, it also does not include bunt singles or bunts in which the batter reached on a fielder’s choice or error, which help to greatly increase run expectancy (I also excluded Nick Swisher’s bunt against Boston where he lost track of the number of outs and bunted on his own). The analysis is limited to successful sacrifice bunts. I’ve also removed all bunts by pitchers, as I think most of us can agree that bunting with an American League pitcher is almost always the correct move.
- We cannot calculate what would have happened if Girardi had chosen not to bunt. To provide an example of why this is an issue, imagine an inning where Brett Gardner bunts a runner over and then Curtis Granderson homers. While we can figure out the run expectancy before and after the bunt and can observe actual runs scored, we can’t know what would have happened if Gardner had not bunted. So if one run was expected and two actual runs were scored, there is still the possibility that without the bunt, three runs would have scored (because Gardner could have reached prior to the home run). If we assume that everything would have been different and Granderson may not have homered had Gardner reached, the expected runs v. actual runs analysis is relevant. As such, this study is making the assumption that the bunt changes the entire inning, such that whatever happened afterward is connected to (but not necessarily caused by) the base/out state created by the bunt. Discarding that assumption does not make the conclusions irrelevant, but it does sap them of some of their power.
Keeping all that in mind, let’s take a look at the sac bunts Girardi called for in 2011.
Brett Gardner
# of sac bunts: 8
Expected runs, before the bunts: 7.0173
Expected runs, after the bunts: 5.4602
Actual runs: 11
Loss of run expectancy: 1.5571
Actual impact: Gain of 3.9827 runs over expected runs
(To be fair to Girardi and his predilection for bunting with Gardner, it is important to note that all of Gardner’s bunts but one came in the late innings of a tight game, when playing for one run is acceptable. The lone exception came against Justin Verlander, which represents another understandable, if not entirely defensible, use of the bunt.)
Eduardo Nunez
# of sac bunts: 6
Expected runs, before the bunts: 5.5296
Expected runs, after the bunts: 4.4064
Actual runs: 3
Loss of run expectancy: 1.1232
Actual impact: Loss of 2.5296 runs under expected runs
Derek Jeter
# of sac bunts: 4
Expected runs, before the bunts: 4.1974
Expected runs, after the bunts: 3.4954
Actual runs: 2
Loss of run expectancy: 0.702
Actual impact: Loss of 2.1974 runs under expected runs
Curtis Granderson
# of sac bunts: 3
Expected runs, before the bunts: 3.7157
Expected runs, after the bunts: 3.2358
Actual runs: 6
Loss of run expectancy: .4799
Actual impact: Gain of 2.2843 runs over expected runs
Ramiro Pena
# of sac bunts: 2
Expected runs, before the bunts: 1.701
Expected runs, after the bunts: 1.3028
Actual runs: 1
Loss of run expectancy: 0.3982
Actual impact: Loss of 0.701 runs under expected runs
One each for Russell Martin, Frankie Cervelli, Chris Dickerson, and Brandon Laird
# of sac bunts: 4
Expected runs, before the bunts: 3.402
Expected runs, after the bunts: 2.6056
Actual runs: 1
Loss of run expectancy: 0.7964
Actual impact: Loss of 2.402 runs under expected runs
Conclusion
# of sac bunts: 27
Expected runs, before the bunts: 25.563
Expected runs, after the bunts: 20.5062
Actual runs: 24
Loss of run expectancy: 5.0568
Actual impact: Loss of 1.563 runs under expected runs
Regarding that actual impact number, I am uncomfortable concluding that the bunts were always directly responsible for what happened after them. For example, I do not think Granderson’s lone “successful” bunt actually caused all 6 runs that subsequently scored in the inning. That said, I think it is fair to conclude that Girardi’s proclivity for bunting did not hurt the Yankees much in 2011. In terms of run expectancy, all of the bunts over the course of the season only cost the Yankees five runs, and that ignores the fact that many of them came in situations where playing for one run at the expense of a big inning is actually the right thing to do. Furthermore, the team outperformed the “runs expected after the bunts,” suggesting that Girardi may have utilized the strategy in optimal situations. Taking into account the fact that the actual runs scored was about the same as the number of runs expected, it seems clear that Joe Girardi’s bunting problem was not much of an detriment to the Yankees in 2011.
Update (12:28 p.m.): I am new to play index, but I just figured out how to get bunt singles and bunt outs listed properly(still no foul bunts, however). Here are the results for the 18 sac bunt attempts that ended without a sac bunt:
10 runners reached base
8 made force outs or popouts
On the outs:
RE before the bunts: 7.5994
RE after the bunts: 4.431
Actual: 7
Loss of RE: 3.1684
Actual impact: 0.5994
On the hits:
RE before the bunts: 9.9545
RE after the bunts: 16.1122
Actual: 17
Loss of RE: Gain of 6.1577
Actual impact: Gain of 7.0455
New total:
RE before the bunts: 43.1169
RE after the bunts: 41.0494
Actual: 48
Loss of RE: Loss of 2.0675 runs
Actual impact: Gain of 4.8831
Leave a Reply
You must be logged in to post a comment.